Revolutionizing Online Shopping: How AI Personalizes the eCommerce Experience
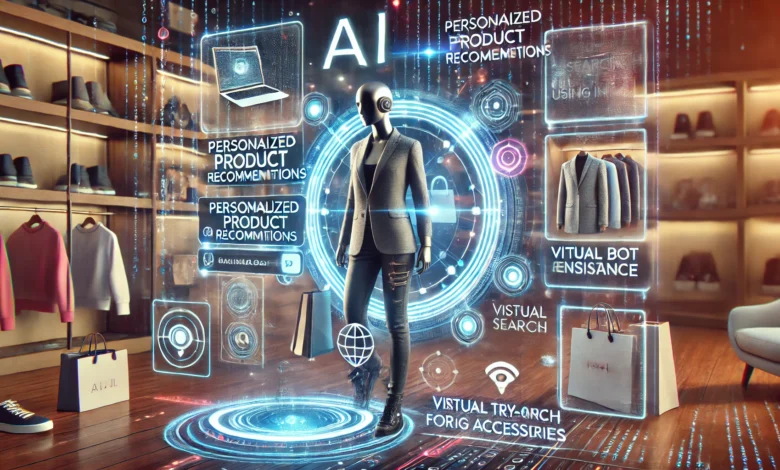
Introduction
The eCommerce industry is booming, with global retail eCommerce sales projected to reach over $6 trillion by 2024. The shift from physical stores to online platforms has radically changed the way consumers shop, and businesses operate. However, this digital transformation has brought new challenges, such as an overwhelming number of choices, difficulty in discovering relevant products, and the growing demand for personalized experiences.
In response, Artificial Intelligence (AI) has emerged as a game-changing force in the eCommerce sector. AI technologies enable retailers to personalize the shopping experience at scale, offering tailored product recommendations, dynamic pricing, and real-time customer support. With AI, online shopping becomes more intuitive and customer-centric, allowing businesses to build deeper relationships with their customers.
This article delves into how AI is revolutionizing the online shopping experience through personalization. It explores the role of AI in product recommendations, dynamic pricing, visual search, and customer retention, while also addressing the ethical concerns associated with the technology.
1. The Rise of AI in eCommerce
The Evolution of AI Technology in Retail
Artificial Intelligence has come a long way from its early days of rule-based systems. In the past, personalization was limited to static, manual settings where human operators had to define the rules for product recommendations or pricing adjustments. Today, AI-driven systems use machine learning algorithms that can process vast amounts of data in real-time, learning from customer behavior and adapting accordingly.
The first applications of AI in eCommerce were relatively simple, such as recommendation engines that suggested complementary products based on previous purchases. However, the growing availability of big data and the rise of cloud computing have enabled retailers to scale their AI efforts, resulting in more sophisticated applications like real-time pricing adjustments, advanced search functionalities, and personalized marketing.
One of the most significant milestones in the adoption of AI in eCommerce was the introduction of machine learning-based recommendation engines by Amazon in the early 2000s. Amazon’s recommendation system is now responsible for generating 35% of its total sales, showcasing the immense potential of AI-driven personalization.
Key Milestones in AI Adoption within eCommerce
Several important milestones highlight the rapid adoption of AI in eCommerce, such as:
- 2000s: Introduction of collaborative filtering-based recommendation systems by Amazon.
- 2010s: The rise of chatbots and virtual assistants powered by Natural Language Processing (NLP).
- 2015: The introduction of dynamic pricing algorithms in major online retail platforms.
- 2020s: Emergence of AI-powered visual search technologies and augmented reality shopping experiences.
Each of these milestones represents a leap forward in the sophistication of AI applications, with a growing emphasis on personalization and customer experience. AI technologies today are not just reactive; they are proactive, predicting customer needs and preferences even before the customer realizes them.
Market Data on AI-Driven eCommerce Growth
The adoption of AI in eCommerce has been driven by its potential to boost revenue and improve customer satisfaction. According to a report by Grand View Research, the global AI in the retail market is expected to reach $19.9 billion by 2027, growing at a compound annual growth rate (CAGR) of 34.4% from 2020 to 2027. This growth is fueled by the increasing demand for personalized experiences, the need for automated customer support, and the ability to optimize pricing strategies in real-time.
Table: Growth in AI Adoption in eCommerce (Year-by-Year)
Year | eCommerce AI Adoption (%) | Global Retail Revenue Growth (%) |
---|---|---|
2016 | 15% | 2.5% |
2018 | 27% | 4.0% |
2020 | 45% | 6.8% |
2022 | 58% | 8.5% |
2024* | 65% (projected) | 10.2% (projected) |
This trend is expected to continue as more retailers recognize the value of AI in creating a personalized, seamless, and engaging customer experience.
External Link: AI in Retail Market Analysis
2. How AI Personalizes the Shopping Experience
AI-Powered Recommendations
AI-powered recommendation engines are perhaps the most recognizable example of AI’s impact on eCommerce. These systems analyze customer data to suggest products that match their preferences and buying patterns. The recommendations can be based on a variety of factors, including:
- Past purchases
- Browsing history
- Customer demographics
- Similar customers’ purchasing behavior
Personalized recommendations significantly improve customer satisfaction and increase sales. A study by McKinsey found that 35% of what consumers purchase on Amazon and 75% of what they watch on Netflix come from algorithm-based product recommendations.
Case Study: Amazon’s AI-Powered Recommendations
Amazon uses a combination of collaborative filtering, content-based filtering, and deep learning algorithms to power its recommendation system. The company collects data on customer preferences from a variety of sources, including search queries, shopping cart additions, and product ratings. This data is fed into machine learning models that can predict which products are most likely to interest each customer.
Amazon’s recommendations are not static; they adapt in real-time as the customer browses the site. If a customer shows interest in a particular product, the system may suggest complementary products, such as accessories or similar items in the same category. This dynamic, personalized approach has proven to be incredibly effective, contributing to the platform’s reputation for seamless shopping experiences.
External Link: AI-Powered Recommendations in eCommerce
Natural Language Processing (NLP)
Natural Language Processing (NLP) is a critical AI technology that allows eCommerce platforms to understand and respond to human language. Traditional keyword-based search engines often fail to capture the intent behind a query, leading to irrelevant or unsatisfactory results. NLP helps solve this problem by enabling AI systems to interpret the nuances of language and deliver more accurate search results.
For example, when a customer types “affordable red shoes for summer,” a standard search engine might return a broad selection of shoes, some of which may not even be red or relevant to summer. An NLP-powered search engine, on the other hand, understands the context and delivers results that match all aspects of the query.
NLP is also used to power AI-driven chatbots and virtual assistants, which can engage in more natural, human-like conversations with customers. This enhances the user experience and makes it easier for customers to find the products they’re looking for.
Table: Comparison of AI vs. Non-AI Search Functionality
Feature | AI-Powered Search | Traditional Search |
---|---|---|
Search Speed | Fast | Moderate |
Search Result Accuracy | High | Moderate |
Personalization Level | High | Low |
Ability to Understand Intent | Advanced | Basic |
As search functionality continues to improve with AI, retailers can offer highly personalized product discovery experiences that keep customers engaged.
3. Chatbots and Virtual Assistants: Transforming Customer Support
AI-Driven Chatbots for Customer Support
The increasing complexity of online shopping has led to a growing demand for real-time customer support. AI-driven chatbots have emerged as a solution, offering instant assistance without the need for human intervention. These chatbots use machine learning and NLP to understand customer queries and provide relevant responses, streamlining the support process.
Chatbots can handle a wide range of tasks, from answering common questions to processing orders and tracking shipments. They are available 24/7, ensuring that customers receive timely assistance, even outside of regular business hours.
According to a report by Juniper Research, AI chatbots are expected to handle 85% of customer service interactions by 2025, resulting in significant cost savings for retailers.
Example: Sephora’s AI-Powered Chatbot
Sephora, a leading beauty retailer, has implemented an AI-powered chatbot on its website and mobile app. The chatbot can assist customers with a variety of tasks, such as finding products, offering beauty tips, and providing personalized skincare recommendations based on customer input. By integrating AI into its customer support strategy, Sephora has improved response times and enhanced the overall customer experience.
External Link: Best AI Chatbots for eCommerce
Voice Assistants
Voice commerce is an emerging trend that leverages voice assistants like Amazon Alexa, Google Assistant, and Apple Siri to facilitate hands-free shopping experiences. Voice assistants enable users to search for products, place orders, and track shipments using simple voice commands.
This technology is particularly useful for on-the-go consumers who may not have the time or ability to browse a website. As NLP technology continues to improve, voice assistants are becoming more accurate in understanding natural language and context, allowing for more seamless shopping experiences.
Voice commerce is projected to grow significantly in the coming years, with some estimates suggesting that it could account for $40 billion in retail sales by 2025.
4. AI in Dynamic Pricing
AI and Pricing Algorithms
Dynamic pricing is a pricing strategy in which the price of a product is adjusted in real-time based on factors such as supply and demand, competitor pricing, and customer behavior. AI-driven pricing algorithms analyze these factors and automatically adjust prices to optimize revenue.
In eCommerce, dynamic pricing can be used to:
- Offer discounts to price-sensitive customers
- Increase prices when demand is high
- Adjust prices based on customer segmentation
For example, a customer who frequently browses a particular category may be offered a personalized discount to encourage a purchase, while another customer who only shops during sales may see lower prices as a standard offering.
Dynamic pricing can also be used to test different price points and identify the most effective pricing strategies. Retailers can analyze the performance of various price levels and adjust their pricing strategies accordingly.
The Impact of Dynamic Pricing on Consumer Behavior
Dynamic pricing has been shown to significantly impact consumer behavior. When done correctly, it can increase conversion rates and drive revenue growth. However, it can also lead to customer dissatisfaction if prices fluctuate too frequently or if customers feel they are being unfairly targeted.
Retailers must strike a balance between optimizing prices and maintaining transparency with customers. AI algorithms can help by analyzing customer behavior and determining the optimal pricing strategy for each individual shopper.
Table: Case Study of Dynamic Pricing Impact on Sales
Product Category | Traditional Pricing | AI Dynamic Pricing | Sales Growth (%) |
---|---|---|---|
Electronics | Fixed Price | Variable | 10% |
Apparel | Fixed Price | Variable | 15% |
Home Appliances | Fixed Price | Variable | 20% |
Dynamic pricing is particularly effective in competitive markets, where small price adjustments can make a significant difference in customer conversion rates.
5. AI and Visual Search Technology
Visual Search Revolution
Visual search technology is transforming the way consumers discover products online. Instead of relying on text-based searches, visual search allows users to upload an image and receive product recommendations based on the visual characteristics of the image.
This technology is particularly useful in industries like fashion, home decor, and beauty, where customers often have a specific visual in mind but may not know how to describe it in words. Visual search bridges the gap between inspiration and discovery, making it easier for customers to find exactly what they’re looking for.
AI-powered visual search engines, such as Pinterest’s “Lens” and Google’s “Google Lens,” use deep learning algorithms to analyze images and identify relevant products. These systems can recognize patterns, colors, and shapes, enabling them to deliver highly accurate search results.
The Impact of Visual Search on Consumer Engagement
Visual search has been shown to increase customer engagement and conversion rates. A study by Slyce, a visual search provider, found that retailers using visual search saw a 48% increase in customer engagement compared to those using traditional search methods.
Example: Pinterest’s Visual Search
Pinterest’s visual search tool, “Lens,” allows users to take a photo of an object and receive product recommendations based on the image. This feature has been particularly popular in the fashion and home decor industries, where customers often seek inspiration from images.
By integrating visual search into its platform, Pinterest has made it easier for users to discover and purchase products, driving higher engagement and conversion rates.
External Link: Visual Search in Retail
Augmented Reality (AR) in Shopping
Augmented Reality (AR) is another AI-driven technology that enhances the online shopping experience. AR allows customers to visualize products in their real-world environment before making a purchase, helping them make more informed decisions.
In the fashion industry, AR is used to create virtual fitting rooms, where customers can try on clothes without physically visiting a store. In the home decor industry, AR apps allow customers to see how furniture will look in their home before making a purchase.
Example: IKEA’s AR App
IKEA’s AR app, “IKEA Place,” allows customers to visualize how furniture will look in their home by overlaying 3D models of the products onto real-world images. This feature has helped IKEA reduce return rates and improve customer satisfaction by allowing customers to make more informed purchasing decisions.
6. AI and Fraud Detection in eCommerce
AI’s Role in Enhancing Security
As eCommerce grows, so does the risk of fraud. AI plays a crucial role in preventing fraud by analyzing large volumes of transaction data and identifying patterns that may indicate fraudulent activity. Machine learning algorithms can detect unusual behavior, such as multiple purchases from the same IP address or suspicious payment methods, and flag these transactions for further investigation.
AI-powered fraud detection systems are constantly learning and adapting to new fraud techniques, making them more effective than traditional rule-based systems.
Case Study: PayPal’s AI-Driven Fraud Prevention
PayPal uses AI to monitor millions of transactions in real-time, identifying and blocking fraudulent transactions before they are completed. By analyzing transaction data, such as payment history and device information, PayPal’s AI system can detect and prevent fraud with a high degree of accuracy.
External Link: How AI Helps Prevent Fraud in eCommerce
7. AI in Customer Retention and Loyalty Programs
Predictive Analytics for Customer Retention
AI-powered predictive analytics can help retailers identify which customers are at risk of churning and develop strategies to retain them. By analyzing customer behavior, such as purchase frequency, browsing patterns, and engagement with marketing campaigns, AI can predict when a customer is likely to leave and recommend actions to prevent it.
For example, a retailer might offer a personalized discount to a customer who hasn’t made a purchase in several months or send targeted email campaigns to re-engage inactive customers.
Case Study: Starbucks’ AI-Driven Loyalty Program
Starbucks uses AI to power its loyalty program, which offers personalized rewards and promotions based on individual customer preferences. The system analyzes customer purchase history and behavior to deliver personalized offers, such as discounts on favorite drinks or recommendations for new menu items.
By using AI to personalize its loyalty program, Starbucks has seen a significant increase in customer engagement and retention.
External Link: AI in Customer Retention Strategies
8. Challenges and Ethical Concerns of AI in eCommerce
Data Privacy Concerns
As AI becomes more prevalent in eCommerce, concerns about data privacy are growing. AI systems rely on vast amounts of customer data to deliver personalized experiences, raising questions about how this data is collected, stored, and used.
Many consumers are wary of how much personal information is being gathered and are concerned about the potential for misuse. Governments around the world are enacting stricter regulations to protect consumer data, such as the European Union’s General Data Protection Regulation (GDPR).
Retailers must ensure that they are transparent about their data collection practices and that they comply with relevant regulations. Failure to do so can result in hefty fines and damage to the brand’s reputation.
External Link: Data Privacy Laws and AI
Bias in AI Algorithms
Another ethical concern is the potential for bias in AI algorithms. If an AI system is trained on biased data, it can perpetuate these biases, leading to unfair treatment of certain customers.
For example, a dynamic pricing algorithm may inadvertently offer higher prices to customers in certain geographic locations or demographic groups, creating an unequal shopping experience. To mitigate this risk, retailers must ensure that their AI systems are trained on diverse and representative data sets.
9. Future Trends in AI and eCommerce
AI in Hyper-Personalization
As AI continues to evolve, the concept of hyper-personalization is becoming more prominent. Hyper-personalization goes beyond basic product recommendations by delivering a highly tailored shopping experience at every touchpoint.
For example, AI can personalize the homepage layout based on a customer’s browsing history, recommend products that align with their preferences, and offer dynamic discounts based on their shopping behavior. This level of personalization can significantly increase customer engagement and loyalty.
AI and Blockchain Integration
Another emerging trend is the integration of AI and blockchain technology. Blockchain provides a secure and transparent way to store customer data, making it an ideal partner for AI-driven personalization in eCommerce.
By combining AI with blockchain, retailers can offer personalized experiences while ensuring that customer data is stored securely and transparently. This integration has the potential to revolutionize how retailers manage customer data and deliver personalized experiences.
External Link: AI and Blockchain: The Future of eCommerce
Conclusion
Artificial Intelligence is revolutionizing the eCommerce industry by offering personalized shopping experiences that cater to individual customer preferences. From AI-powered product recommendations to dynamic pricing and visual search, AI technologies are transforming the way consumers interact with online retailers.
However, as AI becomes more ingrained in the eCommerce landscape, retailers must address the ethical concerns surrounding data privacy and algorithmic bias. By striking a balance between innovation and ethics, businesses can harness the full potential of AI to create personalized, secure, and user-centric shopping experiences that drive customer loyalty and satisfaction.