A Beginner’s Guide to Starting a Dropshipping Business
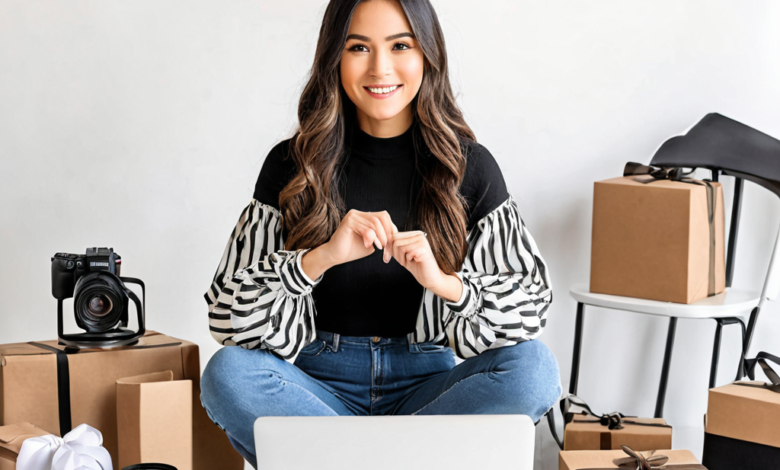
Introduction to Dropshipping
In today’s digital age, the dropshipping business model has gained significant traction as an accessible entry point for aspiring entrepreneurs. Dropshipping allows business owners to sell products directly to consumers without holding any inventory. Instead, when a customer makes a purchase, the seller places an order with a third party, often a wholesaler or manufacturer, who then ships the product directly to the customer. This method streamlines the retail process by minimizing inventory management and logistical challenges.
One of the primary advantages of dropshipping is the low initial investment required to get started. Unlike traditional retail models that necessitate large quantities of stock and warehousing, dropshipping permits entrepreneurs to operate with limited capital. This characteristic makes it particularly attractive to new business owners who may not have substantial financial resources at their disposal. Furthermore, minimal inventory risk is another notable benefit. Since there is no need to purchase products upfront, businesses can avoid the potential losses associated with unsold stock.
Additionally, the flexibility inherent in dropshipping enables entrepreneurs to manage their businesses from virtually any location with an internet connection. This geographical independence is a critical factor contributing to the model’s popularity among digital nomads and those seeking a better work-life balance. The combination of low startup costs, reduced financial risk, and operational flexibility underscores why dropshipping has become an appealing option for many aspiring e-commerce entrepreneurs.
This comprehensive guide is designed to provide you with all the essential steps and insights needed to build a successful dropshipping business. From selecting the right products and suppliers to setting up your online store and marketing effectively, each section of this guide will equip you with the knowledge and tools necessary to navigate the world of dropshipping with confidence and competency.
Researching and Selecting a Niche
Choosing the right niche is paramount to the success of a dropshipping business. A well-selected niche can set the foundation for profitability and sustainability. When researching niches, several key factors should be considered to ensure a well-informed decision: market demand, competition, and personal interest.
Firstly, market demand is crucial. A niche with high demand is likely to attract more customers, leading to higher sales. Tools like Google Trends can be invaluable for gauging interest in particular niches over time, helping to identify trends and seasonal fluctuations. Additionally, keyword planners can reveal the number of searches for specific products, giving insight into potential customer interest levels.
Secondly, evaluating competition is essential. While some level of competition indicates demand, too much can make it challenging to stand out. Analyze competitors by looking at their online presence, product offerings, and customer reviews. This provides valuable insights into what is already available and what gaps might exist in the market.
Finally, personal interest should not be overlooked. Running a dropshipping business requires dedication and passion. Choosing a niche that aligns with personal interests can make the daily tasks more enjoyable and increase the likelihood of long-term success. Personal knowledge and enthusiasm for a niche can also translate into better customer service and marketing efforts.
To conduct thorough market research, start by identifying broad interests and then narrow them down. Utilize tools such as Google Trends to compare the popularity of different niches. Keyword planners can help determine the search volume for specific terms. Other market research tools, like SEMrush and Ahrefs, can provide competitor analysis and further insights into market dynamics.
In summary, selecting a niche is a critical step in starting a dropshipping business. Focus on market demand, assess competition, and consider personal interest. By employing comprehensive market research tools, one can identify niches with the potential for profitability and long-term success.
Finding Reliable Suppliers
One of the fundamental aspects of establishing a successful dropshipping business is sourcing reliable suppliers. These suppliers serve as the backbone of your business, dictating the quality of products, shipping times, and ultimately, customer satisfaction. The process of finding and assessing potential suppliers is crucial and can be facilitated by leveraging well-known directories such as AliExpress, Oberlo, and SaleHoo.
When exploring these directories, the first step is to identify suppliers that align with your niche and business needs. AliExpress is widely known for its vast array of products and suppliers, making it a popular choice for many dropshippers. Oberlo, on the other hand, integrates seamlessly with Shopify stores, allowing for an automated product management experience. SaleHoo provides a comprehensive directory of verified suppliers, ensuring a higher level of trustworthiness.
Once potential suppliers are identified, it is essential to evaluate their reliability rigorously. Key factors to consider include the consistency of product quality, the accuracy of product descriptions, and the efficiency of shipping times. Reliable suppliers should provide high-quality products that meet your standards and are accurately represented in listings. Additionally, prompt and dependable shipping is paramount to maintaining customer satisfaction and minimizing negative reviews.
Evaluating supplier performance can be achieved through several methods. First, examine customer reviews and ratings on the supplier’s platform. These reviews can offer valuable insights into the supplier’s reliability and product quality. Furthermore, consider placing test orders to personally gauge the product quality and shipping efficiency. Communication with the supplier is also critical; responsive and clear communication indicates a professional supplier willing to address concerns promptly.
Be vigilant for red flags when assessing suppliers. Inconsistent communication, an abundance of negative reviews, and unclear return policies can indicate potential issues. When negotiating with suppliers, strive for clarity in terms, such as pricing, shipping times, and return policies. Establishing a mutual understanding in these areas can prevent future disputes and foster a cooperative relationship.
In the realm of dropshipping, the importance of reliable suppliers cannot be overstated. By conducting meticulous research and evaluation, you can build a network of dependable suppliers and lay the groundwork for a thriving dropshipping business.
Setting Up Your Online Store
Establishing your online store is a pivotal step in launching a successful dropshipping business. Begin by selecting an appropriate e-commerce platform. Platforms like Shopify and WooCommerce are popular due to their user-friendly interfaces and comprehensive features. Shopify offers a straightforward setup process and a variety of apps to enhance functionality, whereas WooCommerce integrates seamlessly with WordPress, providing flexibility and extensive customization options.
Choosing a Domain Name
Your domain name is your online identity. Select a unique, memorable, and brand-relevant name. It should be easy to spell and pronounce, avoiding any potential confusion for your customers. Tools like Namecheap can assist in determining the availability of your desired domain. Once you’ve chosen a name, register it promptly to secure your online presence.
Creating a Visually Appealing Website
First impressions matter significantly in e-commerce. Design a visually appealing and user-friendly website. Choose a clean, professional design template that aligns with your brand’s aesthetics. Ensure high-quality images, clear fonts, and consistent color schemes to create a cohesive look. Navigation should be intuitive, allowing customers to find products effortlessly.
Essential E-commerce Features
Incorporating essential e-commerce features is crucial for operational efficiency and customer satisfaction. Integrate secure payment gateways such as PayPal, Stripe, or direct credit card payments to provide multiple payment options for your customers. Utilize SSL certificates to protect customer data and build trust by ensuring their personal information is secure. Mobile responsiveness is non-negotiable; your store should offer a seamless shopping experience across all devices, which aids in capturing a broader audience.
Enhancing Brand and Customer Experience
A strong brand and exceptional customer experience are integral to the success of your dropshipping business. Develop a unique brand identity that resonates with your target audience. This includes an attractive logo, consistent brand messaging, and a compelling value proposition. Prioritize customer experience by offering excellent customer service, hassle-free returns, and prompt responses to inquiries. Personalize interactions to foster loyalty and repeat business.
By meticulously setting up your e-commerce store with these steps, you lay a solid foundation for a thriving dropshipping business.
Creating Your Product Listings
Creating compelling product listings is crucial for attracting and converting visitors to your dropshipping business. The cornerstone of a successful listing lies in high-quality images, which are essential for captivating the attention of potential customers. Ensure your images are clear, well-lit, and showcase the product from multiple angles. Including lifestyle images, which demonstrate the product in real-life settings, can further enhance the appeal.
Equally important is crafting detailed and accurate descriptions. An informative product description should cover all essential features and specifications, addressing potential questions or concerns customers may have. It should highlight the benefits and uses of the product in a way that resonates with your target audience. Clear, succinct language free from jargon can help in conveying the information effectively.
Pricing strategies also play a pivotal role in product listings. Conduct thorough market research to set competitive prices while ensuring they are in sync with your business model and profit margins. Consider using psychological pricing techniques, such as pricing your items just below whole numbers (e.g., $19.99 instead of $20), to make them appear more attractive.
SEO-friendly titles and keywords are vital for improving your product’s visibility in search engine results. Incorporate relevant keywords naturally within the product title and description without making it seem forced. This practice not only helps in better ranking on search engines but also assists customers in finding your products more easily.
To stand out from competitors, emphasize your unique selling points (USPs). This could be anything from exceptional customer service, a unique feature of your product, or an exclusive offer. Clearly highlight these USPs in your listings to give customers a compelling reason to choose your product over others. Additionally, regularly monitoring and updating your listings based on feedback and market trends can further refine and enhance their effectiveness.
Implementing these elements cohesively can significantly improve the quality of your product listings, making them more attractive and effective in converting visitors into loyal customers.
Marketing Your Dropshipping Store
Effective marketing strategies are crucial for driving traffic and generating sales for your dropshipping store. One of the most popular methods is online advertising, with platforms such as Google Ads, Facebook Ads, and Instagram Ads offering targeted reach to potential customers. These platforms allow you to define your audience based on demographics, interests, and behaviors, ensuring your ads are seen by those most likely to convert. Bid strategically and monitor your ad performance to make data-driven decisions.
In addition to paid advertising, content marketing is a powerful tool for attracting organic traffic. Developing high-quality blog posts, product guides, and how-to articles can establish your store as a valuable resource. By integrating targeted keywords naturally into your content, you can improve your search engine optimization (SEO) and rank higher in search results. Regularly updating your blog with fresh content will maintain engagement and improve your SEO performance over time.
Leveraging influencer partnerships can also boost your store’s visibility. By collaborating with influencers who align with your brand, you can tap into their audience for increased exposure. Ensure the influencers you work with have a genuine following and high engagement rates. Sponsored posts, product reviews, and giveaways can generate buzz and provide social proof to potential customers.
Social media promotion is essential for creating brand awareness and engaging with your audience. Maintain an active presence on platforms relevant to your target market, such as Instagram, Facebook, Twitter, or TikTok. Regularly posting engaging content, sharing user-generated content, and running promotions can help build a loyal community around your brand. Utilize analytics tools to track engagement and adjust your strategy accordingly.
Email marketing remains one of the most effective ways to convert leads into customers. Building an email list allows you to send personalized content, promotions, and updates directly to your subscribers. Segment your list to tailor your messages and include compelling calls to action to boost conversions. Automating your email campaigns can save time and ensure consistent communication with your audience.
Finally, always monitor and track the performance of your marketing campaigns closely. Utilize analytics to gain insights into what works and what doesn’t. Continually optimize your strategies based on these insights to improve your return on investment and achieve better results for your dropshipping business.
Handling Orders and Customer Service
In the dropshipping business model, the order processing workflow is integral to maintaining smooth operations and ensuring customer satisfaction. The process begins when a customer places an order on your online store. Immediately upon receiving this order, the information is forwarded to the respective supplier. This can be done through automated systems that communicate directly with suppliers’ databases or via manual methods such as email or a supplier’s app.
Once the supplier receives the order, they will pick, pack, and ship the product directly to your customer. It is essential to continuously monitor the order status during this phase, ensuring that the supplier ships the products on time and provides tracking information. Keeping track of delivery timelines and updating the customer about their order status via email notifications or your store’s portal enhances transparency and trust.
Providing excellent customer service in a dropshipping business is pivotal for retaining customers and building a positive reputation. To achieve this, clear communication is key. Always be prompt in responding to customer inquiries and handling their concerns. A well-structured FAQ section on your website can preemptively address common queries, easing the customer service workload.
Managing returns and refunds requires a well-defined policy that is easily accessible to customers. Work closely with your suppliers to ensure that any returns are processed smoothly, and refunds or replacements are handled professionally. An organized return process, coupled with courteous service, can turn a potentially negative experience into a positive one.
Setting clear and realistic expectations is another vital strategy. Provide accurate product descriptions, estimated shipping times, and clear policies regarding returns and refunds. These steps help manage customer expectations and reduce the likelihood of dissatisfaction. Additionally, addressing common issues swiftly and efficiently demonstrates your commitment to customer satisfaction.
The significance of maintaining good customer relations cannot be overstated in a dropshipping business. Positive experiences often translate into repeat business and valuable word-of-mouth advertising. By prioritizing timely order processing and excellent customer service, you not only meet but exceed customer expectations, fostering loyalty and driving long-term success.“`html
Scaling and Optimizing Your Dropshipping Business
Once you have successfully established your dropshipping business, the next crucial step is scaling it for growth and optimizing operations to maintain profitability. One of the primary strategies for scaling is expanding your product offerings. By introducing new products that align with your brand and target audience, you can attract a broader customer base and enhance revenue streams. Proper market research and staying updated on industry trends will enable you to select high-potential products.
Automation is another significant aspect of optimizing your dropshipping business. Implementing tools for inventory management, order processing, and customer service can reduce manual interventions, allowing you to handle a larger volume of orders efficiently. For example, utilizing inventory management software can help keep track of stock levels and prevent overselling, while chatbots can provide instant support to customers.
Improving website performance is also vital for scaling your business. A fast, user-friendly website can enhance the customer shopping experience and lead to higher conversion rates. Ensure your website is mobile-friendly, has quick load times, and features an intuitive navigation system. Regularly test and update the site to fix any issues that could hinder user experience.
Leveraging analytics is crucial for making informed decisions. Tools like Google Analytics can provide insights into customer behavior, sales trends, and website performance. By analyzing this data, you can identify areas for improvement and optimize marketing strategies. Furthermore, understanding metrics such as customer acquisition cost (CAC) and customer lifetime value (CLV) can help in planning long-term growth strategies.
Enhancing customer lifetime value (CLV) is essential for sustained profitability. Strategies such as upselling and cross-selling can increase the average order value and encourage repeat purchases. Additionally, fostering strong relationships with customers through loyalty programs, personalized communication, and excellent customer service can boost retention rates.
Finally, staying updated with industry trends and continually optimizing operations is key to remaining competitive. Regularly review and adjust your strategies based on market changes, customer feedback, and technological advancements. This proactive approach will ensure your business not only scales but also remains profitable in the dynamic e-commerce landscape.